Efficient keyword search over graph-structured data based on minimal covered r-cliques
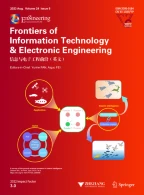
Keyword search is an alternative for structured languages in querying graph-structured data. A result to a keyword query is a connected structure covering all or part of the queried keywords. The textual coverage and structural compactness have been known as the two main properties of a relevant result to a keyword query. Many previous works examined these properties after retrieving all of the candidate results using a ranking function in a comparative manner. However, this needs a time-consuming search process, which is not appropriate for an interactive system in which the user expects results in the least possible time. This problem has been addressed in recent works by confining the shape of results to examine their coverage and compactness during the search. However, these methods still suffer from the existence of redundant nodes in the retrieved results. In this paper, we introduce the semantic of minimal covered r-clique (MCCr) for the results of a keyword query as an extended model of existing definitions. We propose some efficient algorithms to detect the MCCrs of a given query. These algorithms can retrieve a comprehensive set of non-duplicate MCCrs in response to a keyword query. In addition, these algorithms can be executed in a distributive manner, which makes them outstanding in the field of keyword search. We also propose the approximate versions of these algorithms to retrieve the top-k approximate MCCrs in a polynomial delay. It is proved that the approximate algorithms can retrieve results in two-approximation. Extensive experiments on two real-world datasets confirm the efficiency and effectiveness of the proposed algorithms.
This is a preview of subscription content, log in via an institution to check access.
Access this article
Subscribe and save
Springer+ Basic
€32.70 /Month
- Get 10 units per month
- Download Article/Chapter or eBook
- 1 Unit = 1 Article or 1 Chapter
- Cancel anytime
Buy Now
Price includes VAT (France)
Instant access to the full article PDF.
Rent this article via DeepDyve
Similar content being viewed by others
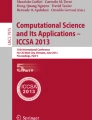
An Effective Keyword Search Method for Graph-Structured Data Using Extended Answer Structure
Chapter © 2013

Key-core: cohesive keyword subgraph exploration in large graphs
Article 06 August 2021
Finding smallest k-Compact tree set for keyword queries on graphs using mapreduce
Article 24 March 2015
Change history
References
- Bergamaschi S, Guerra F, Interlandi M, et al., 2013. QUEST: a keyword search system for relational data based on semantic and machine learning techniques. Proc VLDB Endowm, 6(12): 1222–1225. https://doi.org/10.14778/2536274.2536281ArticleGoogle Scholar
- Bergamaschi S, Guerra F, Interlandi M, et al., 2016. Combining user and database perspective for solving keyword queries over relational databases. Inform Syst, 55: 1–19. https://doi.org/10.1016/j.is.2015.07.005ArticleGoogle Scholar
- Bron C, Kerbosch J, 1973. Finding all cliques of an undirected graph. Commun ACM, 16(9): 575–577. https://doi.org/10.1145/362342.362367ArticleMATHGoogle Scholar
- Calado P, da Silva AS, Laender AHF, et al., 2004. A Bayesian network approach to searching Web databases through keyword-based queries. Inform Process Manag, 40(5): 773–790. https://doi.org/10.10167/j.ipm.2004.03.002ArticleGoogle Scholar
- Dasari NS, Ranjan D, Mohammad Z, 2014. Maximal clique enumeration for large graphs on Hadoop framework. Proc 1 st Workshop on Parallel Programming for Analytics Applications, p.21–30. https://doi.org/10.1145/2567634.2567640
- de Virgilio R, Cappellari P, Miscione M, 2009. Cluster-based exploration for effective keyword search over semantic datasets. In: Laender AHF, Castano S, Dayal U, et al. (Eds.), Conceptual Modeling — ER 2009. Springer Berlin Heidelberg, p.205–218. https://doi.org/10.1007/978-3-642-04840-1_17ChapterGoogle Scholar
- Ding BL, Yu JX, Wang S, et al., 2007. Finding top-k min-cost connected trees in databases. IEEE 23 rd Int Conf on Data Engineering, p.836–845. https://doi.org/10.1109/ICDE.2007.367929
- Ghanbarpour A, Naderi H, 2018. A model-based keyword search approach for detecting top-k effective answers. Comput J, 62(3): 377–393. https://doi.org/10.1093/comjnl/bxy056ArticleMathSciNetGoogle Scholar
- Golenberg K, Kimelfeld B, Sagiv Y, 2008. Keyword proximity search in complex data graphs. Proc ACM SIGMOD Int Conf on Management of Data, p.927–940. https://doi.org/10.1145/1376616.1376708
- Guo L, Shao F, Botev C, et al., 2003. XRANK: ranked keyword search over XML documents. Proc ACM SIGMOD Int Conf on Management of Data, p.16–27. https://doi.org/10.1145/872757.872762
- Hao YF, Cao HP, Qi Y, et al., 2015. Efficient keyword search on graphs using MapReduce. IEEE Int Conf on Big Data, p.2871–2873. https://doi.org/10.1109/BigData.2015.7364106
- He H, Wang HX, Yang J, et al., 2007. BLINKS: ranked keyword searches on graphs. Proc ACM SIGMOD Int Conf on Management of Data, p.305–316. https://doi.org/10.1145/1247480.1247516
- Kargar M, An AJ, 2011. Keyword search in graphs: finding r-cliques. Proc VLDB Endowm, 4(10): 681–692. https://doi.org/10.14778/2021017.2021025ArticleGoogle Scholar
- Kargar M, An AJ, 2015. Finding top-k r-cliques for keyword search from graphs in polynomial delay. Knowl Inform Syst, 43(2): 249–280. https://doi.org/10.1007/s10115-014-0736-0ArticleGoogle Scholar
- Kargar M, An AJ, Yu XH, 2014. Efficient duplication free and minimal keyword search in graphs. IEEE Trans Knowl Data Eng, 26(7): 1657–1669. https://doi.org/10.1109/TKDE.2013.85ArticleGoogle Scholar
- Kim S, Lee W, Arora NR, et al., 2012. Retrieving keyworded subgraphs with graph ranking score. Expert Syst Appl, 39(5): 4647–4656. https://doi.org/10.1016/j.eswa.2011.08.136ArticleGoogle Scholar
- Kimelfeld B, Sagiv Y, 2006. Finding and approximating top-k answers in keyword proximity search. Proc 25 th ACM SIGMOD-SIGACT-SIGART Symp on Principles of Database Systems, p.173–182. https://doi.org/10.1145/1142351.1142377
- Lawler EL, 1972. A procedure for computing the K best solutions to discrete optimization problems and its application to the shortest path problem. Manag Sci, 18(7): 401–405. https://doi.org/10.1287/mnsc.18.7.401ArticleMathSciNetMATHGoogle Scholar
- Le TN, Bao FZ, Ling TW, 2015. Exploiting semantics for XML keyword search. Data Knowl Eng, 99: 105–125. https://doi.org/10.1016/j.datak.2015.06.003ArticleGoogle Scholar
- Li GL, Ooi BC, Feng JH, et al., 2008. EASE: an effective 3-in-1 keyword search method for unstructured, semi-structured and structured data. Proc ACM SIGMOD Int Conf on Management of Data, p.903–914. https://doi.org/10.1145/1376616.1376706
- Liu F, Yu C, Meng WY, et al., 2006. Effective keyword search in relational databases. Proc ACM SIGMOD Int Conf on Management of Data, p.563–574. https://doi.org/10.1145/1142473.1142536
- Mass Y, Sagiv Y, 2012. Language models for keyword search over data graphs. Proc 5 th ACM Int Conf on Web Search and Data Mining, p.363–372. https://doi.org/10.1145/2124295.2124340
- Mesquita F, da Silva AS, de Moura ES, et al., 2007. LABRADOR: efficiently publishing relational databases on the web by using keyword-based query interfaces. Inform Process Manag, 43(4): 983–1004. https://doi.org/10.1016/jipm.2006.09.018ArticleGoogle Scholar
- Nguyen K, Cao JL, 2012. Top-K data source selection for keyword queries over multiple XML data sources. J Inform Sci, 38(2): 156–175. https://doi.org/10.1177/0165551511435875ArticleGoogle Scholar
- Ning XM, Jin H, Jia WJ, et al., 2009. Practical and effective IR-style keyword search over semantic web. Inform Process Manag, 45(2): 263–271. https://doi.org/10.1016/j.ipm.2008.12.005ArticleGoogle Scholar
- Pan YF, Wu YQ, 2013. ROU: advanced keyword search on graph. Proc 22 nd ACM Int Conf on Information & Knowledge Management, p.1625–1630. https://doi.org/10.1145/2505515.2505743
- Park CS, Lim S, 2015. Efficient processing of keyword queries over graph databases for finding effective answers. Inform Process Manag, 51(1): 42–57. https://doi.org/10.1016/jipm.2014.08.002ArticleGoogle Scholar
- Park J, Lee SG, 2011. Keyword search in relational databases. Knowl Inform Syst, 26(2): 175–193. https://doi.org/10.1007/s10115-010-0284-1ArticleGoogle Scholar
- Qin L, Yu JX, Chang LJ, et al., 2009. Querying communities in relational databases. Proc IEEE 25 th Int Conf on Data Engineering, p.724–735. https://doi.org/10.1109/ICDE.2009.67
- Sagharichian M, Naderi H, Haghjoo M, 2015. ExPregel: a new computational model for large-scale graph processing. Concurr Comput Pract Exp, 27(17): 4954–4969. https://doi.org/10.1002/cpe.3482ArticleGoogle Scholar
- Segundo PS, Lopez A, Batsyn M, et al., 2016. Improved initial vertex ordering for exact maximum clique search. Appl Intell, 45(3): 868–880. https://doi.org/10.1007/s10489-016-0796-9ArticleGoogle Scholar
- Segundo PS, Artieda J, Strash D, 2018. Efficiently enumerating all maximal cliques with bit-parallelism. Comput Oper Res, 92: 37–46. https://doi.org/10.1016/j.cor.2017.12.006ArticleMathSciNetMATHGoogle Scholar
- Tomita E, Tanaka A, Takahashi H, 2006. The worst-case time complexity for generating all maximal cliques and computational experiments. Theor Comput Sci, 363(1): 28–42. https://doi.org/10.1016/j.tcs.2006.06.015ArticleMathSciNetMATHGoogle Scholar
- Wang D, Zou L, Zhao DY, 2015. Top-k queries on RDF graphs. Inform Sci, 316: 201–217. https://doi.org/10.1016/j.ins.2015.04.032ArticleMATHGoogle Scholar
- Xu YW, Guan JH, Li FR, et al., 2013. Scalable continual top-k keyword search in relational databases. Data Knowl Eng, 86: 206–223. https://doi.org/10.1016/j.datak.2013.03.004ArticleGoogle Scholar
- Yu T, Liu MC, 2017. A linear time algorithm for maximal clique enumeration in large sparse graphs. Inform Process Lett, 125: 35–40. https://doi.org/10.1016/jipl.2017.05.005ArticleMathSciNetMATHGoogle Scholar
- Yuan Y, Wang GR, Chen L, et al., 2013. Efficient keyword search on uncertain graph data. IEEE Trans Knowl Data Eng, 25(12): 2767–2779. https://doi.org/10.1109/TKDE.2012.222ArticleGoogle Scholar
Author information
Authors and Affiliations
- School of Computer Engineering, Iran University of Science and Technology, Tehran, 13114-16846, Iran Asieh Ghanbarpour, Khashayar Niknafs & Hassan Naderi
- Department of Computer Engineering, University of Sistan and Baluchestan, Zahedan, 98167-45845, Iran Asieh Ghanbarpour
- Asieh Ghanbarpour
You can also search for this author in PubMed Google Scholar
You can also search for this author in PubMed Google Scholar
You can also search for this author in PubMed Google Scholar
Contributions
Asieh GHANBARPOUR completed the proofs and mathematical parts, drafted the manuscript, and revised it. Khashayar NIKNAFS completed the algorithms, carried out the implementations, and evaluated the results. Hassan NADERI guided the research and supervised the writing of the manuscript.
Corresponding author
Additional information
Compliance with ethics guidelines
Asieh GHANBARPOUR, Khashayar NIKNAFS, and Hassan NADERI declare that they have no conflict of interest.